Getting started with Full-Text Search (part 2 of 5)
A Tutorial: How a Simple Full-Text Search Could Work—Based on an Example
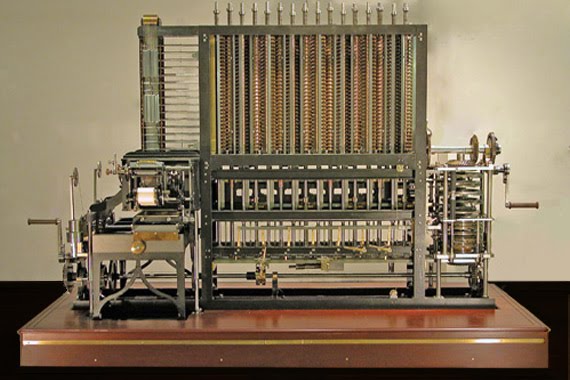
Continuation. Previous part can be found here - Getting started with Full-Text Search (part 1 of 5).
Tip
|
The sources for this part are available to you at https://github.com/esurovtsev/getting-started-with-fulltext-search/tree/part-2. |
Brute-force Search
All right. We know how to get a list of all of the document identifiers (file names), as well as how to fetch each document’s contents by its identifier. It seems that now we finally have everything we need to start working on the actual search.
But, let’s stop for a few moments to think: how can we actually do the searching?
The straightforward way might look something like this:
-
Take each file from the list, one by one;
-
Search for the user’s query string in the file contents;
-
If the query string is found, then put the file name in the list of positive results;
-
At the end, return the list of all positive results as the search outcome.
Since the user’s search request is a string of text and the contents of the documents are also plain text, this approach of comparing strings should work. But how should we implement it?
First of all, we will need a new service that will be responsible for all search-related operations. And just so you know, over time we will be accumulating various methods for performing searches as these methods come to mind as we move forward with our project.
At this point, however, we will simply create the first method in our service. We will call it findUsingBruteForce
, since that is exactly what it will do. In light of steps that will follow, we will add the suffix _simple
to the method’s name. That way, when we improve the algorithm and create another, more advanced, method we will be able to distinguish this function from other similar functions.
@Service
class SearchService(private val documentService: DocumentService) {
fun findUsingBruteForce_simple(request: String): List<String> =
documentService
.findAllIds() // (1)
.filter { docId ->
documentService
.findById(docId) // (2)
?.let { docContent -> docContent.contains(request) } // (3)
?: false
}
}
-
Get a list of all documents.
-
Load content for each document from the list.
-
If the content is loaded, check that it contains the user’s search query by simple string comparision.
As you can readily see, the implementation is quite straightforward. We request a list of all document IDs, and then load each document—one by one in sequence—and check to see if it contains the search string. After processing them all, we can return a list of document IDs that contain the search string supplied in the query.
Note
|
Files vs. Documents If you’ve been reading this tutorial carefully, you may have noticed that we often use different terms for referencing the same thing. For example, sometimes we might say |
To actually perform our search, we will still need to create a new command.
enum class SearchType {
BRUTE_FORCE
}
@ShellMethod("Performs a search request on the documents")
fun search(
@ShellOption(help = "Defines a search type") type: SearchType,
@ShellOption(help = "Defines a search query") query: String
): List<String> =
when (type) {
SearchType.BRUTE_FORCE ->
addHeader(searchService.findUsingBruteForce_simple(query))
}
private fun addHeader(result: Collection<String>): List<String> =
listOf("Results found: ${result.size}")
.plus("---------------------------------------")
.plus(result)
.plus("---------------------------------------")
Now, let’s run the command and take a look at its output.
Tip
|
When typing commands in the shell, you might want to try a very useful feature called autocompletion. Just press the <Tab> key when you are in the middle of entering a predefined command or value. |
shell:>help search
NAME
search - Performs a search request on the documents
SYNOPSYS
search [--type] search-type [--query] string
OPTIONS
--type search-type
Defines a search type
[Mandatory]
--query string
Defines a search query
[Mandatory]
shell:>search --type BRUTE_FORCE --query "glass mountain"
Results found: 1
---------------------------------------
The Iron Stove.txt
---------------------------------------
shell:>
Great! Our algorithm works and even returns a single document in the result set.
But let’s see if this will satisfy us.
When submitting the query “glass mountain”, we expected to get a list of all documents where it speaks in some way about glass mountain or, again (in technical language), this probably should be a text where both of these words (i.e., glass and mountain) are used.
Is it true, then, that there is only one document in the whole database that meets our criteria?
Obviously not! There should be more documents.
Just take a look. The most obvious example, “The Glass Mountain.txt” document, was not found at all by our search.
We definitely need to fix that!
Nevertheless, despite the imperfections of our first algorithm, and a somewhat disappointing outcome, this method does demonstrate one of the basic concepts of search technologies. This concept is known as Boolean Search.
Note
|
Boolean Search (by definition of IT Law Wiki) is a search methodology based on Boolean mathematics. In Boolean searches, an ‘and’ operator between two words or other values (for example, ‘pear AND apple’) means one is searching for documents containing both of the words or values, not just one of them. An ‘or’ operator between two words or other values (for example, ‘pear OR apple’) means one is searching for documents containing either of the words. |
In practice, Boolean Search means that, in our search code, we have defined a Boolean predicate, and for each document, we can define whether this predicate is satisfied or not. Accordingly, all documents that satisfy the given Boolean predicate are included in the results returned by the search, and those that do not satisfy the predicate are omitted.
Tokenization
Now, returning to our program, you recall that we are not fully satisfied with our search results. We need to do something to fix that. So, let’s think: what could be the reason for our results falling short of our expectations?
We know that we have several documents that, by all indications, should be included in our result set. Yet, for some reason, these documents were omitted. For example, the explicit candidate “The Glass Mountain.txt” was not included in our results list.
So, let’s take a look at the contents of this document to figure out what the problem might be.
Sticking his spurs into his horse he made a rush at the mountain, and got up half-way, then he calmly turned his horse’s head and came down again without a slip or stumble. The following day he started in the same way; the horse trod on the glass as if it had been level earth, and sparks of fire flew from its hoofs. All the other knights gazed in astonishment, for he had almost gained the summit, and in another moment he would have reached the apple-tree; but of a sudden a huge eagle rose up and spread its mighty wings, hitting as it did so the knight’s horse in the eye.
Upon reviewing the contents, we can see that one of the potential problems could be that the words from our search query do not always occur together in sequence in this story. Sometimes the search terms are separated by other words, or in the story they may appear in a different order from that of our input search terms.
In our present method, when we search the document for our entire search query string, it does not work for all cases according to our expectations. Clearly, we need more flexible search criteria to obtain our desired results.
What, then, do we need to do?
Here’s an idea: we could split our search request into separate words, which we will call tokens (where the whole process of dividing text into separate token terms is called tokenization). Then we can proceed to verify if each token is actually in the contents of each document.
Note
|
Tokenization is the process of demarcating and, possibly, classifying sections of a string of input characters. The resulting tokens are then passed along for additional processing. The tokenization process can be considered a subtask of parsing input parameters. |
In order to perform our tokenization, let’s create a new component that will be responsible for the preliminary processing of user requests. We will name it TokenAnalyzer. This component, when called, will delegate tokenization tasks to individual (smaller) components, depending upon how, exactly, we need to split strings into tokens.
It also makes sense to remove duplicate tokens, should they be created by our process. For example, it seems clear that, for our use-case, the two requests “glass mountain” and “glass mountain glass” are virtually identical. (Be aware that this may be an incorrect assumption for other similar use-cases, however.)
@Component
class TokenAnalyzer {
fun analyze_whitespaceTokenizing(input: String): Collection<String> =
WhitespaceTokenizer.tokenize(input).toSet()
}
object WhitespaceTokenizer {
private val whitespace = Regex("\\s+")
fun tokenize(input: String): Collection<String> =
input.split(whitespace)
}
Let’s see how our new Tokenizer splits our request string. To accomplish this, however, we need to create a new search method and apply an update for the existing search command.
fun findUsingBruteForce_tokenize(request: String): Collection<String> {
val terms = analyzer.tokenize(request)
return documentService
.findAllIds()
.filter { docId -> documentService
.findById(docId)
?.let { it.containsAllTerms(terms) } ?: false }
}
fun String.containsAllTerms(terms: Collection<String>): Boolean =
terms
.map { contains(it) }
.fold(true) { result, element -> result && element }
fun search(...) {
...
SearchType.BRUTE_FORCE_TOKEN ->
addHeader(searchService.findUsingBruteForce_tokenize(query))
...
}
Now, let’s try it to see what we get.
shell:>search --type BRUTE_FORCE_TOKEN --query "glass mountain"
Results found: 4
---------------------------------------
The Glass Mountain.txt
The Glass Axe.txt
Thumbelina.txt
The Iron Stove.txt
---------------------------------------
shell:>
Great! This time our results look better. And what is especially satisfying is that the document “The Glass Mountain.txt”, which we were expecting, is now included in our result set.
Direct Index
Okay. Now we have some search results to review.
But, just as we did before, let’s stop for a moment to consider if there are any additional flaws in our current solution.
There is one flaw, and I think the answer lies in the words, “brute force.”
In our present search, we must load the contents of each document one-by-one, then search the contents of each document, for each search term (token). For our list of 56 small stories, this is not really a big deal. The results still are returned almost instantly.
But imagine how this approach might work in a real-life situation where the list of stories might reach millions or even billions of items. And the stories themselves, instead of being short, one-page texts, might be full-fledged works containing a novel or even an entire book the size of Leo Tolstoy’s War and Peace. It is obvious that, in such a case, our “brute force” search would be stuck for a long, long time in execution.
Let’s try to solve this problem and accelerate execution of the search.
The first idea that comes to mind is to reduce the volume of data that the process must handle during execution. We will take the same approach as we did with our brute-force search with one exception: we will work, not with the document itself, but only with its tokens. Clearly, in this case, the set of all document tokens should be significantly smaller than the entire contents of the document—especially since we will have no duplicates in our token set.
This approach also gives us another huge advantage: it should reduce the time required to determine if the document matches our search query.
Just think about this: comparing two sets of sorted tokens can be accomplished much faster than searching for the occurrences of each token in the whole contents of a document.
So then, what do we need to implement such a method?
For starters, we must determine the location for storing the indexed results in the form of tokens. Let’s create a new configuration item for keeping this data.
com.searching-bits.get-started-fulltext-search.direct-index-dir: target/classes/direct-index
Additionally, our configuration component should reflect our required new settings…
fun getDirectIndexDir(): String = getAllProperties()[DIRECT_INDEX_DIR_KEY] ?: ""
And since the application is now responsible for the directory with its indexed data, the application also should take responsibility for creating the directory itself, if the required directory does not exist.
@PostConstruct
fun init() {
listOf(getDirectIndexDir()).forEach {
val dir = File(it)
if (!dir.exists() || !dir.isDirectory) {
dir.mkdirs()
}
}
}
Let’s validate that our configuration works as we expect.
shell:>config
com.searching-bits.get-started-fulltext-search.data-dir: target/classes/stories
com.searching-bits.get-started-fulltext-search.direct-index-dir: target/classes/direct-index
shell:>exit
➜ getting-started-with-fulltext-search > ls target/classes
META-INF application.yaml banner.txt com direct-index stories
Wonderful! We have everything in place. Let’s now get down to content indexing. For this we will create a new component with the name DirectIndexer.
@Component
class DirectIndexer(
private val analyzer: TokenAnalyzer,
private val documentService: DocumentService,
private val config: Configuration
) {
fun createIndex() {
// delete old index
File(config.getDirectIndexDir()).listFiles()?.forEach { it.delete() }
// generate new index
documentService.findAllIds().forEach { docId ->
documentService.findById(docId)?.let {
File("${config.getDirectIndexDir()}/$docId")
.writeText(analyzer
.analyze_whitespaceTokenizing(it)
.joinToString("\n"))
}
}
}
}
Also, as usual, we will need a new shell command. This time it will be a command for starting the new indexing process we just created.
enum class IndexType {
DIRECT
}
@ShellMethod("Generates a search Index")
fun createIndex(
@ShellOption(help = "Defines an index type") type: IndexType
): String {
when (type) {
IndexType.DIRECT -> directIndexer.createIndex()
}
return "Index created: $type"
}
And, at last, we generate the index using our new command.
shell:>create-index --type DIRECT
Index created: DIRECT
shell:>
If we compare the size of all the stories and the size of the direct index we just created, it will be very clear that the volume of data to be processed during our search will decrease to less than half its original volume (the raw data being about 820KB, and the newly created index being about 360KB). That’s a very meaningful improvement!
Next, let’s see what the resulting index actually looks like.
>cat direct-undex/The Crow.txt
THE
CROW(13)
(13)
From
the
Polish.
Kletke.
Once
upon
a
time
…
In order to work with our newly created index, however, we need to add a couple of new functions to our previously created DirectIndexer. Replicating the approach we used previously for the DocumentService, we will need two methods: 1) get a list of indexed documents and 2) get the contents of the index for a given document.
fun findAllIds(): List<String> =
File(config.getDirectIndexDir()).listFiles().map { it.name }
fun findById(documentId: String): List<String> =
this
.findAllIds()
.findLast { it == documentId }
?.let { File("${config.getDirectIndexDir()}/$it") }
?.takeIf { it.exists() && it.isFile && it.canRead() }
?.let { it.readLines() }
?: listOf()
At this point, we have all the necessary components in place, and we can finally write a new search method that will employ our new index capabilities.
fun findUsingDirectIndex(request: String): Collection<String> {
val terms = analyzer.tokenize(request)
return documentService
.findAllIds()
.filter { docId -> directIndexer
.findById(docId)
?.let { docTokens -> docTokens.containsAll(terms) } }
}
As you can probably see, this new method is very similar to the previous “FindUsingBruteForce_tokenize”. The only real difference is that we do not check the contents of the documents. Instead we check against the search query tokens found in the token set of each document.
Let’s update the command for this search method now so we can give it a try.
fun search(...) {
...
SearchType.DIRECT_INDEX -> addHeader(searchService.findUsingDirectIndex(query))
...
}
shell:>create-index --type DIRECT
Index created: DIRECT
shell:>search --type BRUTE_FORCE_TOKEN --query "glass mountain"
Results found: 4
---------------------------------------
The Glass Mountain.txt
The Glass Axe.txt
Thumbelina.txt
The Iron Stove.txt
---------------------------------------
shell:>search --type DIRECT_INDEX --query "glass mountain"
Results found: 2
---------------------------------------
The Glass Mountain.txt
The Iron Stove.txt
---------------------------------------
shell:>
As you can see, the search works. But, for reasons yet unknown to us, this search returns even fewer results than our earlier brute-force method. Let’s take a look. We need to figure out what went wrong this time.
Look at the document “Thumbelina.txt”, one of the documents not found in our “direct-index” case. Let’s analyze its index.
>cat direct-undex/Thumbelina.txt
…
he
were
made
of
glass;
he
had
the
mountains,
great
mountains
where
snow
…
Plainly, the “glass” and “mountain” tokens that we need are missing from the index for this document. Instead, we find “glass;”, “mountains,”, “mountains”, and other similar terms. (Did you notice the inclusion of the punctuation marks and plural forms in the tokens for this document?)
Suddenly it seems clear as to why everything worked on this document using the full-text brute-force search method but is not working using our latest “direct-index” method. In our minds, it seems logical that the value “glass” is part of “glass;”, which gave us the search hit when using the “brute-force” method. However, when comparing tokens in our new “direct-index” method, these expected results are excluded.
It also seems apparent that our system, for the same reason, is not able to determine that “mountain” (singular) should logically correspond to “mountains” (plural).
We need to improve the process of preparing tokens somehow. And this is what we are going to do next. In coming chapters, we will be applying improvements-one by one—to our search algorithm.
Note
|
The process we are going to work on next is called Normalization. Breaking text into tokens if only half of what is required. To make those tokens more search-ready, they need to go through a normalization process to remove insignificant differences between otherwise identical values. Another important term here is Analysis. This is a process of tokenization and normalization applied at the same time. |
Onward, then. With that general background, we are going to start working on a new Analyzer component for our search engine application. We will get started on it in the next chapter.
Continue reading - Getting started with Full-Text Search (part 3 of 5).