Getting started with Full-Text Search (part 3 of 5)
A Tutorial: How a Simple Full-Text Search Could Work—Based on an Example
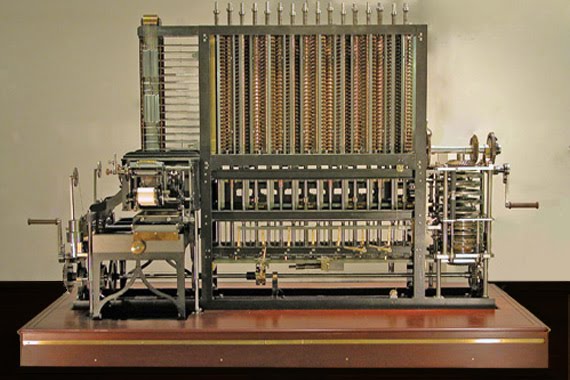
Continuation. Previous part can be found here - Getting started with Full-Text Search (part 2 of 5).
Tip
|
The sources for this part are available to you at https://github.com/esurovtsev/getting-started-with-fulltext-search/tree/part-3. |
Improving Analyzer: Better Tokenizer
We already have a few ideas for improving our search. But where should we begin?
Let’s try to create a better process for token creation. Having reviewed our previous results, it is very clear that splitting into tokens simply based on whitespaces does not give us what we really need.
If we take a look at the generated index, it is obvious that many of our tokens have “garbage” data included, such as punctuation marks, dashes, brackets, and more. Each of these is preventing us from working with the tokens effectively and, as a result, we fail to obtain the search results we are expecting.
>cat direct-undex/A Story about a Darning-needle.txt
...
fine!'
‘It
is
all
right!'
Fingers,
seizing
sha'n't
her
her--shavings
round
waist.
‘Look,
coming
with
my
train!'
as
drew
long
thread
after
her;
sealing-wax.'
but
...
We will try to fix our tokenization process, but we will take the existing WhitespaceTokenizer
as the basis for our changes and improve it from there.
Our first step will be to collect all the typical tokenization issues we have uncovered in the review of our existing index. Then, based on this data set, we will create “by-example” rules for our unit testing. Once our unit tests are successful, we can work on a real implementation of the whole process.
class BetterTokenizerTest {
@Test
fun tokenize() =
listOf(
"‘Take" to arrayOf("Take"),
"tight!'" to arrayOf("tight"),
"‘Don't" to arrayOf("Don"),
"right!'" to arrayOf("right"),
"Darning-needle." to arrayOf("Darning", "needle"),
"Fingers;" to arrayOf("Fingers"),
"breast-pin!'" to arrayOf("breast", "pin"),
"sealing-wax.'" to arrayOf("sealing", "wax"),
"sha'n't" to arrayOf("sha"),
"her--shavings," to arrayOf("her", "shavings"),
"‘'Fingers.''" to arrayOf("Fingers"),
"Dip-into-everything," to arrayOf("Dip", "into", "everything"),
"can't--it" to arrayOf("can", "it"),
"egg-shell." to arrayOf("egg", "shell"),
"lie." to arrayOf("lie")
).forEach {
assertThat(underTest.tokenize(it.first))
.containsExactlyInAnyOrder(*it.second)
}
}
Now, implementing our better tokenizer.
@Component
class BetterTokenizer(private val whitespaceTokenizer: WhitespaceTokenizer) {
private val nonAlpha = Regex("[^a-zA-Z]")
fun tokenize(input: String): Collection<String> =
whitespaceTokenizer
.tokenize(nonAlpha.replace(input, " "))
.filter { it.length > 1 }
}
Of course, in order to use these changes, we must propagate them up the chain to the command level. This means we will need a new method for TokenAnalyzer
.
fun analyze_betterTokenizing(input: String): Collection<String> =
BetterTokenizer.tokenize(input).toSortedSet()
We also must implement a new function for creating another direct index with our better tokenization. (To do so, let’s just copy those parts from the old method that does almost the same thing.)
fun createBetterIndex() {
// delete old index
File(config.getDirectIndexDir()).listFiles()?.forEach { it.delete() }
// generate new index
documentService.findAllIds().forEach { docId ->
documentService.findById(docId)?.let {
File("${config.getDirectIndexDir()}/$docId")
.writeText(analyzer.analyze(it).joinToString("\n"))
}
}
}
And, of course, we need the option to create a new index type with a new command.
enum class IndexType {
DIRECT, BETTER_DIRECT
}
fun createIndex(...) {
...
IndexType.BETTER_DIRECT -> directIndexer.createBetterIndex()
...
}
Since we have now changed the way in which we perform the tokenization of document contents, we also need to apply the same tokenization method for our search queries. And this is a very important point!
Note
|
When doing full-text searches, we want tokens of the search request to have precisely the same form as tokens from the documents. |
fun findUsingBetterDirectIndex(request: String): Collection<String> {
val terms = analyzer.analyze(request)
return documentService
.findAllIds()
.filter { docId -> directIndexer
.findById(docId)
?.let { docTokens -> docTokens.containsAll(terms) }}
}
Lastly, we will need to define a new type of search for our app.
fun search(...) {
...
SearchType.BETTER_DIRECT_INDEX ->
addHeader(searchService.findUsingBetterDirectIndex(query))
...
}
Note
|
For instructional purposes, we are interested in preserving all our steps in the source code. Therefore, we do not modify the existing methods. Instead we are constantly creating new functions, leaving the old functions still available in the form that we originally created them. This makes our codebase a bit messy, but very effective for our learning along the way. In real life, of course, it makes much more sense not to duplicate existing methods while making small changes. Instead, existing code would be refactored when new requirements arise. |
Even though we have already created unit tests to assure the correctness of our new tokenization algorithm, let’s go ahead and take a look at the new output in our “better” index. This will provide us with visual “proof” that our new process works as we expect.
>cat direct-undex/A Story about a Darning-needle.txt
...
all
right
seizing
round
waist
Look
coming
with
my
train
as
drew
long
thread
after
...
Okay. Compared to the previous version’s output, we can plainly see that significant progress has been made.
Although the new process of tokenization now meets our requirements, we could still add a couple of improvements that would increase further the quality of the final processed tokens. We will embed new functionality into our already implemented analyze_betterTokenizing()
method. Then it will be available immediately from our already existing shell commands.
Improving Analyzer: Case Insensitive Search
The next thing we could improve in our search engine would be to bring all the tokens to the same register. The fact is the case of words in the search query may not match the case of the otherwise logically equal words in the contents of the documents. What’s more, if we look again at our index, even there we have several variations of the same token differing only by case. Our human mind makes us think of them as the same word, but they appear to our system as separate, logically independent terms.
THE GLASS MOUNTAIN(16) (16) From the Polish. Kletke. Once upon a time there was a Glass Mountain at the top of which stood a castle made of pure gold, and in front of the castle there grew an apple-tree on which there were golden apples.
Clearly, our present search is entirely case-sensitive. For example, if the user enters queries as “glass mountain”, “Glass Mountain”, or “GLASS MOUNTAIN”, then the results will be different for each case. But this is not the behavior that our users will likely expect to see. So, we need to set our documents’ contents to lowercase (for our search) and request tokens that are “equal” (also in lowercase).
Note
|
In practice it does not matter if we use lowercase tokens or uppercase tokens. The important point is that we need to normalize them and make them of the same case. We do lowercase just because it happened somehow to become the common way to normalize tokens in the “real” world. |
All right. Let’s do this. We will make the change by adding our first filter LowerCaseFilter
to the Analysis process chain.
interface Filter { // (1)
fun filter(input: String): Collection<String> // (2)
}
@Component
class LowerCaseFilter : Filter {
override fun filter(input: String): List<String> =
listOf(input.toLowerCase())
}
fun TokenAnalyzer.analyze_betterTokenizing(input: String): Collection<String> =
BetterTokenizer
.tokenize(input)
.toSet()
.flatMap { lowerCaseFilter.filter(it) }
.toSortedSet()
-
At the end we would need several filters in place so we define a common filter’s interface.
-
Filter takes a token and returns list of tokens as a result of ‘filtering’. The resulting list can contain token itself if no filtering needed, be empty if the token was filttered out, or contain several other tokens as a result of the source token expansion.
Improving Analyzer: Stop Words
The next improvement we might want to include in our search engine would be adding stop-word processing.
What is that?
Let’s take a look at the index we just generated in our last round. Do you notice that it contains a lot of common words that carry no semantic meaning for our search at all? Do you see words such as “or”, “and”, “as”, “for”, “be”, “you”, “I”, and more? These words are common in our language and, thus, will appear in virtually every document.
At the same time, these words are so common that they do not characterize the documents themselves, since they do not determine its semantic content. We call these stop-words because we really don’t need to search for them, and they can significantly interfere with obtaining our desired search results.
For example, a query for “you are” will almost certainly return all the documents to our search results. This is not at all helpful. Therefore, the stop-word concept is to ignore such words when indexing and performing searches. We simply stop searching for them—stop-words.
Note
|
Stop words (by definition of Computer Hope) are commonly used words that are excluded from searches to help index and parse web pages faster. Some examples of stop words are: ‘a’, ‘and’, ‘but’, ‘how’, ‘or’ and ‘what’. While the majority of all Internet search engines utilize stop words, they do not prevent a user from using them, but they are ignored. For example, if you were to search for “What is a motherboard?”, the search engine would only look for the term ‘motherboard’. |
In order to implementation of stop-words, we will begin by storing a list of stop-words in a text file. Each row in the file will contain one word. To do this, we will need to create a list of the words to be included and set up a configuration component to return the location of our stop-words file in our file system.
com.searching-bits.get-started-fulltext-search.stop-words: target/classes/stopwords.txt
const val STOP_WORDS_FILE_KEY = "com.searching-bits.get-started-fulltext-search.stop-words"
fun getStopWordsFile(): String =
getAllProperties()[STOP_WORDS_FILE_KEY] ?: "stopwords.txt"
Note
|
It is quite logical to assume that stop-words depend on the user’s language and, for English, we definitely would have one list of potential stop-words, while for German or Spanish there will be completely different sets of such words. If for some reason you find you need a more complete set of words, you can Google such a list for yourself. There are many examples of such lists, including the one from Google itself. |
an
about
ah
and
are
as
at
be
can
cannot
did
didn
do
does
…
Adding a new filter comes next.
@Component
class StopwordsFilter(val config: Configuration) : Filter {
private lateinit var stopWords: Set<String>
@PostConstruct
fun init() {
stopWords = File(config.getStopWordsFile()).readLines().toSet()
}
override fun filter(input: String): Collection<String> =
if (stopWords.contains(input)) listOf() else listOf(input)
}
And, of course, we must not forget to update the analysis process chain with the newly created filter.
fun TokenAnalyzer.analyze_betterTokenizing(input: String): Collection<String> =
betterTokenizer
.tokenize(input)
.toSet()
.flatMap { lowerCaseFilter.filter(it) }
.flatMap { stopwordsFilter.filter(it) }
.toSortedSet()
Improving Analyzer: Stemming
Another potential problem with using tokens is that the words for which we are searching may appear in the documents in different forms. The most common example of this is plural versus singular forms. For instance, the query token “mountain” will never match the document token “mountains” even though they are virtually identical in our minds.
Fortunately, however, this issue is well known already and there is a solution for it. It’s called Stemming.
Note
|
In linguistic morphology and information retrieval, stemming is the process of reducing inflected (or sometimes derived) words to their word stem, base or root form—generally a written word form. The stem needs not be identical to the morphological root of the word; it is usually sufficient that related words map to the same stem, even if this stem is not in itself a valid root. |
So, we need to apply the stemming process in our search engine in order to reduce inflectional and, sometimes, derivationally related forms of a word to a common base form of the word. For example:
“am”, “are”, “is” → “be”
“car”, “cars”, “car’s”, “cars’” → “car”
The result of such mapping of text will be something like this:
“the boy’s cars are different colors” → “the boy car be differ color”
How can we accomplish this in practice?
Generally speaking, stemming is based on a deep linguistic analysis of a particular language, and the implementation of a good algorithm could easily be the topic of a major PhD work. Additionally, it is obvious that stemming for every language should be based on its own unique algorithm affected by word formations and the rules of each particular language.
As a result, it would be a bit problematic for us to create something of real value for effective stemming within the scope of this short writing. Nevertheless, why don’t we just create a small workaround that, perhaps, demonstrates the basic concepts of stemming?
To do this we will implement some simplified transformation rules to reduce the words to their roots. We will use rules that are simple and based on our inner sense of language. Then we can see what results we get.
The algorithm should work like this: we define a list of typical, frequently used word ending and cut them off the ends of our tokens. We assume, in doing this, that we will get the root of the word. (We recognize that, in practice, this is not the case, but for our demonstration purposes, it will suffice.) And, since the word endings may take several forms (such as “s” and “es” for plurals), we will have our rule assume that the cutting operation that results in the shortest token should be considered the correct stemming rule to apply.
@Component
class StemmingFilter : Filter {
class SuffixRule(private val suffix: String) {
fun stemming(input: String): String? =
if (input.endsWith(suffix))
input.substring(0, input.length - suffix.length).ifEmpty { null }
else null
}
private val rules: List<SuffixRule> = listOf(
SuffixRule("ed"),
SuffixRule("s"),
SuffixRule("ing"),
SuffixRule("e"),
SuffixRule("ly"),
SuffixRule("ty"),
SuffixRule("ability"),
SuffixRule("ness"),
SuffixRule("er"),
SuffixRule("e"),
SuffixRule("es"),
)
override fun filter(input: String): Collection<String> =
listOf(rules
.mapNotNull { it.stemming(input) }
.fold(input) { result, element ->
if (result.length < element.length) result else element })
}
And, as usual, we need to include the newly created StemmingFilter in our analyzer chain.
fun TokenAnalyzer.analyze_betterTokenizing(input: String): Collection<String> =
betterTokenizer
.tokenize(input)
.toSet()
.flatMap { lowerCaseFilter.filter(it) }
.flatMap { stopwordsFilter.filter(it) }
.flatMap { stemmingFilter.filter(it) }
.toSortedSet()
.toList()
We’ve made a lot of changes so far, so let’s stop here and see how our new results set looks.
shell:>create-index --type BETTER_DIRECT
Index created: BETTER_DIRECT
shell:>
All right! It looks like the index is exactly what we wanted.
back
bear
beat
becaus
becom
been
befor
believ
bend
birth
bit
black
born
both
bottl
bow
box
boy
break
...
Great. Now let’s try running our initial search query using the old alongside the new methods to see the differences in the results.
shell:>search --type BRUTE_FORCE_TOKEN --query "glass mountain"
Results found: 4
---------------------------------------
The Glass Mountain.txt
The Glass Axe.txt
Thumbelina.txt
The Iron Stove.txt
---------------------------------------
shell:>search --type BETTER_DIRECT_INDEX --query "glass mountain"
Results found: 4
---------------------------------------
The Glass Mountain.txt
The Glass Axe.txt
Thumbelina.txt
The Iron Stove.txt
---------------------------------------
shell:>
Wow! We finally got the same results as we had from the brute-force search at the beginning!
Continue reading - Getting started with Full-Text Search (part 4 of 5).