Getting started with Full-Text Search (part 4 of 5)
A Tutorial: How a Simple Full-Text Search Could Work—Based on an Example
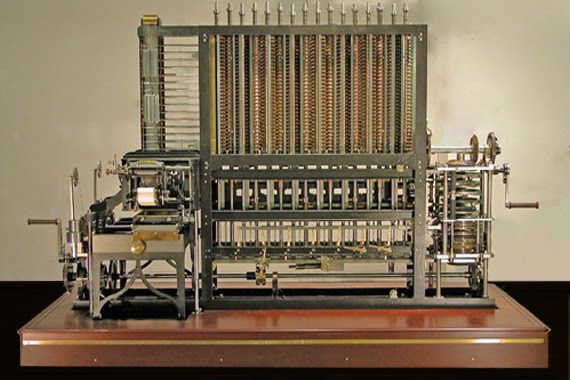
Continuation. Previous part can be found here - Getting started with Full-Text Search (part 3 of 5).
Tip
|
The sources for this part are available to you at https://github.com/esurovtsev/getting-started-with-fulltext-search/tree/part-4. |
Improving Analyzer: Synonyms
Everything seems great—sort of. Our search works. The results are as expected. But we find ourselves at the same place we were when we used our crude brute-force method. We get the same results we had previously when we submit the same query. Let’s try to take this another step forward by improving the behavior of our system further.
But what ideas should we try next?
Consider that the user submitting the request may not know the precise way to formulate the request in order to get the best results. We tend to make mistakes or type less-than-perfect queries.
For example: what would our present search system find if we submit a “glass peak” query?
shell:>search --type BETTER_DIRECT_INDEX --query "glass peak"
Results found: 0
---------------------------------------
---------------------------------------
shell:>
Nothing was found. But in this case our lack of results is quite understandable. None of the documents contain the “peak” token. On the other hand, we might assume that the user’s intent was to search for “glass mountain”, or that, at least, there was some expectation for a similar outcome.
As you might guess, to solve this problem, we can enrich our tokens with synonyms.
To do this, we will add a new appropriate filter to the process chain.
@Component
class SynonymsFilter : Filter {
val synonyms = mapOf(
"mountain" to listOf(
"hill",
"bluff",
"cliff",
"elevation",
"peak",
"pile",
"ridge",
"sierra",
"volcano")
)
override fun filter(input: String): Collection<String> =
synonyms[input]?.plus(input) ?: listOf(input)
}
fun TokenAnalyzer.analyze_betterTokenizing(input: String): Collection<String> =
betterTokenizer
.tokenize(input)
.toSet()
.flatMap { lowerCaseFilter.filter(it) }
.flatMap { stopwordsFilter.filter(it) }
.flatMap { stemmingFilter.filter(it) }
.flatMap { synonymsFilter.filter(it) }
.toSortedSet()
Here are a couple of things you should know about synonyms:
We can improve the quality of our search by “teaching” the system how to better understand the meanings of different abbreviations and acronyms. But the question remains: what is the best way to include all possible synonyms in our search engine? After all, there are millions of them to be considered.
Unfortunately, the answer is: there is no such way. The good news, however, is that you simply do not need to do it.
It is very rare, indeed, that you will need to create a search engine that knows “everything about everything.” Usually our tasks are quite limited to a specific domain of knowledge.
How should we proceed then?
By studying users’ behavior, we can, eventually, get an idea of the possible correlations between different terms supplied by the system’s users. These correlations then become the basis for, and define, our “synonyms.”
Even large “factory-size” search engines work in much the same way. In one way or another, the search engine must determine the knowledge domain that your search query matches. (At least all really good search engines try to accomplish this.)
For example: how could we enrich the acronym AUS? What’s that?
Is it “Atlantic University Sport” or “Advanced Upper Stage” or “Advanced Underwriting Service” or, perhaps, just a reference to “Australia”? so, we need to know the knowledge domain of the users in order to properly answer this question.
shell:>create-index --type BETTER_DIRECT
Index created: BETTER_DIRECT
shell:>search --type BETTER_DIRECT_INDEX --query "glass peak"
Results found: 4
---------------------------------------
The Glass Mountain.txt
The Glass Axe.txt
Thumbelina.txt
The Iron Stove.txt
---------------------------------------
shell:>
Still, there are a couple of challenges we should mention here, even though we will not solve them in the scope of this writing:
First, keep in mind that the order of the filters is very important! For example, if we first start stemming, then the data that our synonyms filter gets will already be stemmed. These will not likely be the best data for our synonyms filter to apply.
In such a case, the synonyms filter would provide synonyms for already modified (stemmed) words. These may lack the full range of values that the synonyms filter requires to do its best work. Furthermore, if we decide to get rid of the stemming process, or simply change it (a little or entirely), it is likely we would need to adapt our synonyms filter, as well. This kind of logic leads to making our system fragile (where a small change in one component leads to many changes across the whole system).
Additionally, think about what we must do if, somewhere in the middle of our development, we realize that the list of synonyms is not complete or correct. What must be done if we need to change it?
In the case of a search query, it’s pretty simple. We would just change the algorithm, and the next time a user submits a new query, we have updated query results.
But what should we do with the document index?
The only way to change it is to rebuild the entire index for all the documents.
And what if you no longer have access to all the documents, or the documents are available, but there are so many (or they are so large) that re-indexing takes hours or even days? How then would we continue with a system that is already in production?
Anticipating these challenges, there appears to be only one way to proceed. We need to enrich our data with synonyms only for the search queries. This is fairly easy, relatively painless, and does not affect the index itself. This is how most search engines work.
However, this is not so easy to implement in our current use-case. We have an ‘AND’ predicate—remember? This means that all tokens must be present in the document in order to match our query. So, in the scope of this writing, we will keep doing the “wrong” thing: we will enrich both our search query and the document index with synonyms. We just urge you to remember that this is for simplicity here, but it is not a best practice for most production environments.
Inverted Index
We now have an outcome that satisfies us. But, as is all too usual, there is one thing that does not work perfectly.
Think about this for a moment: how do we search?
Yes. We have an index to use for collecting tokens. But, when we search, we are still required to iterate through each index one by one. So, if there were millions of documents, we would have to step through millions of index files. This is significant overhead and will not lead to good results in a production environment.
And to make things worse, imagine if we were to transfer our current search technique into an arena of physical objects. How would we search for information in an ordinary paper book?
Our search would look like this: we would open the first page and look for “glass mountain” on that page. Then we would turn the page and search again, line by line, trying to catch a glimpse of the “glass mountain” words. We would then repeat this process for each of the remaining 856 pages. Finally, we will find all of the pages where the requested “mountain” appears.
Clearly, something is wrong with this approach. Don’t you agree?
And there is a better way to find what we need—at least if we’re still talking about paper books. We can just open the book’s Index and search for “glass mountain”. Almost immediately we will have the list of page numbers where “glass mountain” is found in the book.
Now, compare the amount of time we would spend using the first method, going through the book page by page, with the amount of time we would spend doing the same search using the book’s index. This approach of using the book’s Index has taken its own place in search technologies under the name Inverted Index.
Note
|
Inverted index is a structure, which is designed to allow very fast full-text searches. An inverted index consists of a list of all the unique words that appear in any document, and for each word, a list of the documents in which it appears. |
So, the concept of an inverted index is that, for any term, we can instantly get a list of document IDs that contain the term. And, if our search query contains several terms, then the method will provide lists of document IDs separately for each term. We can then get an intersection of all the separate lists to give us a single concise list of the documents that satisfy our query.
Now it should be plainly obvious that working with the lists of document identifiers is much easier and less intensive than iterating through all the documents or all their indices.
Consider this example. We have three documents:
-
five jellyfish sitting on a rock
-
the ants go marching three by three
-
five cheeky monkeys jumping on the bed
Then our inverted index would look like this:
Term | Documents | Term | Documents | Term | Documents | |||
---|---|---|---|---|---|---|---|---|
a |
{ 1 } |
ants |
{ 2 } |
bed |
{ 3 } |
|||
by |
{ 2 } |
cheeky |
{ 3 } |
five |
{ 1, 3 } |
|||
go |
{ 2 } |
jellyfish |
{ 1 } |
jumping |
{ 3 } |
|||
marching |
{ 2 } |
monkeys |
{ 3 } |
on |
{ 1, 3 } |
|||
rock |
{ 1 } |
sitting |
{ 1 } |
the |
{ 2, 3 } |
Note
|
Please note that, in this example, we only look at how the inverted index works. We intentionally skip all additional processing, such as stemming, removing stop words, and so forth. |
Using this inverted index, if the user enters the query “on the five”, then we must perform the following operations:
-
Find lists of document IDs for each term:
on { 1, 3 }
,the { 2, 3 }
,five { 1, 3 }
-
Intersect the lists:
{ 1, 3 }
⩄{ 2, 3 }
⩄{ 1, 3 }
={ 3 }
-
The resulting list would contain document IDs that satisfy the request:
{ 3 }
We see, then, that the query “on the five” matches only one document from the list: ID 3 – “The five cheeky monkeys jumping on the bed”.
Note
|
Typically, each inverted index element consists of the term itself plus the list of document identifiers called a Postings List. In live systems, postings lists can contain millions, or even billions, of document IDs. So, in practice, various techniques are used for merging postings lists quickly. For example, the term could contain the frequency with which it occurs in the content. This allows us to optimize the intersection routine by setting the order of merging lists. |
There we have it. A brief introduction to the concepts of Inverted Index operations.
Now, let’s make something real. We will employ concepts of the inverted index in our application.
First of all, we will need to store the inverted index somewhere. Let’s start by adding the configuration for that.
com.searching-bits.get-started-fulltext-search.inverted-index-file: target/classes/inverted-index.txt
const val INVERTED_INDEX_FILE_KEY = "com.searching-bits.get-started-fulltext-search.inverted-index-file"
@Value("\${$INVERTED_INDEX_FILE_KEY}")
private lateinit var invertedIndexFile: String
For demonstration purposes, we will keep the generated index as a text file in JSON format. That way, if necessary, we can easily analyze any possible problems that may arise regarding it. An element of inverted index could look like this:
{ "six": ["The Six Swans.txt", "Mr. Lismore and the Widow.txt"] }
As you can see, it consists of the term itself along with a postings list with document IDs (still remember that filename is a document ID in our system?).
In order to work with the index, we need to introduce a new component, which will also be responsible for generating the inverted index.
@Component
class InvertedIndexer(
private val analyzer: TokenAnalyzer,
private val documentService: DocumentService,
private val config: Configuration
) {
private val gson = GsonBuilder().setPrettyPrinting().create()
private val index: MutableMap<String, MutableList<String>> = mutableMapOf() // (1)
fun createIndex() {
// delete old index
File(config.getInvertedIndexFile()).delete() // (2)
index.clear()
// generate new index
documentService.findAllIds().forEach { docId -> // (3)
documentService.findById(docId)?.let { doc -> // (4)
analyzer
.analyze_betterTokenizing(doc)
.map { it to docId } // (5)
.forEach { // (6)
index[it.first]?.add(it.second)
?: index.put(it.first, mutableListOf(it.second))
}
}
}
File(config.getInvertedIndexFile()).writeText(gson.toJson(index)) // (7)
}
}
-
The contents of the inverted index is stored in memory and should be loaded at startup.
-
Before creating a new index, the old one should be deleted and cleared from RAM.
-
Get a list of all documents for indexing.
-
Get the content of the document by its ID.
-
Group a term and a document ID into pair of values.
-
Put each pair into the corresponding place in the inverted index.
-
Save the inverted index on the disk, so it can be loaded the next time the application starts.
We also need to make sure that when the application starts, the inverted index is automatically loaded.
@PostConstruct
fun loadIndex() {
val invertedIndex = File(config.getInvertedIndexFile())
if (invertedIndex.exists() && invertedIndex.isFile && invertedIndex.canRead()) {
index.putAll(gson.fromJson(invertedIndex.readText(), MutableMap::class.java)
as MutableMap<String, ArrayList<String>>)
}
}
To create an inverted index, we will also need a new command.
fun createIndex(...) {
...
IndexType.INVERTED -> invertedIndexer.createIndex()
...
}
So, we’re ready! Let’s generate the inverted index and see what it looks like.
shell:>create-index --type INVERTED
Index created: INVERTED
{
"six": [
"The Six Swans.txt",
"Mr. Lismore and the Widow.txt",
"The Invisible Prince.txt",
"The Witch and her Servants.txt",
"The Story of King Frost.txt",
"How Six Men travelled through the Wide World.txt",
"The Dead Wife.txt",
"Minions of the Moon.txt",
"Blockhead Hans.txt"
],
"swan": [
"The Six Swans.txt",
"The Witch and her Servants.txt",
"The Steadfast Tin-soldier.txt"
]
}
Good. It looks as we expected.
Now, let’s use it for performing the search queries. To do this, however, we need a method that will return a postings list for a given term.
fun InvertedIndexer.getPostingListByToken(token: String): List<String> =
index[token] ?: listOf()
We will also need the appropriate search method to perform intersection of the postings lists.
fun SearchService.findUsingInvertedIndex(request: String): Collection<String> =
analyzer
.analyze_betterTokenizing(request)
.map { invertedIndexer.getPostingListByToken(it).toSet() }
.reduce { a, b -> a.intersect(b) }
And, to test the method, we will specify a new shell command.
fun search(...) {
...
SearchType.INVERTED_INDEX ->
addHeader(searchService.findUsingInvertedIndex(query))
...
}
Now, let’s see how it works.
shell:>search --type BETTER_DIRECT_INDEX --query "glass volcano"
Results found: 4
---------------------------------------
The Glass Mountain.txt
The Glass Axe.txt
Thumbelina.txt
The Iron Stove.txt
---------------------------------------
shell:>search --type INVERTED_INDEX --query "glass volcano"
Results found: 4
---------------------------------------
The Glass Mountain.txt
The Glass Axe.txt
Thumbelina.txt
The Iron Stove.txt
---------------------------------------
As you can see, it provides the same outcome as the direct index method we implemented earlier. So, we can say that our inverted index idea works!
Continue reading - Getting started with Full-Text Search (part 5 of 5).