Getting started with Full-Text Search (part 5 of 5)
A Tutorial: How a Simple Full-Text Search Could Work—Based on an Example
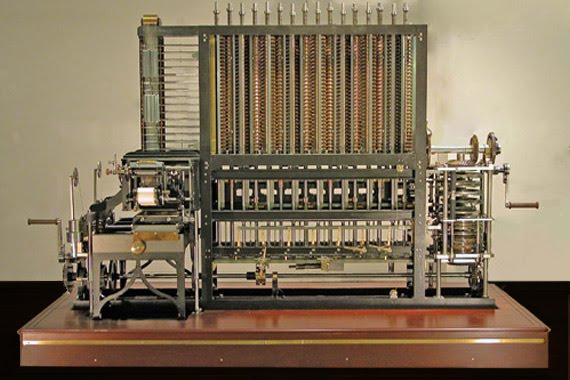
Continuation. Previous part can be found here - Getting started with Full-Text Search (part 4 of 5).
Tip
|
The sources for this part are available to you at https://github.com/esurovtsev/getting-started-with-fulltext-search. |
Matching Query
Now let’s consider another matter related to our search algorithm. Imagine that we wanted to find a story but, because we read it some time ago, we can only remember a very basic outline of the tale. As a result, we can only formulate a very vague search query like, “Well, it was something really interesting about a massive glass hill,” or some statement equally ambiguous.
Let’s try searching for this story.
shell:>search --type INVERTED_INDEX --query "massive glass hill"
Results found: 0
---------------------------------------
---------------------------------------
Hmmmm… Nothing was found.
We probably need to simplify our query, leaving only the words most relevant to our search. And what would be relevant here?
Let’s try searching using “massive hill”.
shell:>search --type INVERTED_INDEX --query "massive hill"
Results found: 0
---------------------------------------
---------------------------------------
Nothing again! All right, then let’s try “glass hill”.
shell:>search --type INVERTED_INDEX --query "glass hill"
Results found: 5
---------------------------------------
The Glass Mountain.txt
Minions of the Moon.txt
The Glass Axe.txt
Thumbelina.txt
The Iron Stove.txt
---------------------------------------
Finally! Okay. It seems the results are better this time. But remember, we had to think and reformulate our query in order to fit it to the capabilities of our system. That’s not good in terms of our users’ experience.
Is there a way we can improve the behavior of our system one more time?
Let’s think about how our search is proceeding presently: we verify that all query terms are in the document. But that’s not always the case. If the document contains only a part of the query (for example, only “glass hill”, as in our case) we still would like to see it in our result set.
There is always a chance that there would be no documents that strictly match our search query. But the best strategy, it would seem, would be to offer some results—meaning documents that at least are a partial fit to our query—instead of returning an empty result set.
In order to fix the current behavior, we will need to improve our algorithm in such a way that if the document contains at least one occurrence of a search token, then we assume that the document satisfies our query. To do this, we will create a new search method that still employs the existing inverted index.
fun findUsingGreedyInvertedIndex(request: String): Collection<String> =
analyzer
.analyze(request)
.map { invertedIndexer.getPostingListByToken(it).toSet() }
.reduce { a, b -> a.plus(b) }
fun search(...) {
...
SearchType.GREEDY_INVERTED_INDEX ->
addHeader(searchService.findUsingGreedyInvertedIndex(query))
...
}
Let’s see how it works.
shell:>search --type GREEDY_INVERTED_INDEX --query "massive glass hill"
Results found: 27
---------------------------------------
The Glass Mountain.txt
The Nightingale.txt
Mr. Lismore and the Widow.txt
The Story of Big Klaus and Little Klaus.txt
The Wrong Black Bag.txt
The Little Green Frog.txt
Minions of the Moon.txt
Blockhead Hans.txt
The Glass Axe.txt
The Magic Ring.txt
...
All right! We have our results, including the “Glass Mountain” story we needed. Unfortunately, a new problem has appeared. You may have noticed that there are many more documents in our results set than would be necessary to satisfy our case. (In fact, we have 27 items, which constitute half our entire database of documents!)
This happened because all the documents in which at least one search term was present ended up in our results. So, it is logical. But working with such a large list probably makes us uneasy because, frankly, we don’t know where to start when presented with such a huge list.
Relevance
It seems the problem we have just encountered is that we find too many documents in our search results. What is not clear, however, is what to do about such a large list.
Imagine for a moment that we are working with a real production system and that several thousands of documents are returned by the query. How do we find those “three” that you need amidst such a large number of documents?
One approach would be to sort them based on how closely the documents in your result set correspond to the search criteria submitted. Then, perhaps, the users can check only a few documents at the top of the list to find what they want. We can even choose to hide some of the documents, for instance, and show only the top ten documents. Alternatively, we could display the documents page by page, allowing users to decide if they need to see additional results.
In order to correctly sort the documents, however, we will need to use an appropriate sorting algorithm—that is to say, we will need to properly rank them.
Note
|
Relevance is the ability to rank results based on how relevant they are to the specific query submitted. |
Typically, content relevance is calculated based on complex logic that may include many factors and conditions, which may also be related to system specifics. Among the many factors taken into consideration when calculating relevance would be the frequency with which the search term(s) appear in each document’s content; if the search terms are near to each other in the documents, possibly forming a phrase; or if the search term(s) in some way characterize some documents’ content (for example: if the frequency of a search term is high in one document but not present, or very sparsely present, in all other documents); and other factors as well.
Also, please keep in mind that knowing the general search domain provides other important factors that may be applied. By way of example, if a user likes certain categories, sites, or topics, these factors can be used to better apply relevance to the search results. Similarly, if the documents are news articles, then the publication date may also be a significant factor in determining relevance for the user.
As a general rule, search engines already contain common mathematical algorithms for calculating relevance and displaying results in accordance with the relevance calculations. So, at the outset, you may not need to know much about how the relevance is being calculated.
For our example, we will implement a naïve and rather simplistic algorithm. We will assume that the greater the count of query terms present in the document, the higher the document’s relevance to the user. Accordingly, the higher the relevance rating for the document, the higher in the list of returns it should be placed for the user.
To do this, we need to know how many times each token appears in each document. And to maintain backward compatibility with our older search methods, we will not change the structure of the inverted index. Instead, we will simply duplicate tokens within the index itself.
If you are thinking that this method of storing information is not necessarily the most rational, you are correct. However, for ease of implementation and for demonstrating our concepts, we will employ this suboptimal approach.
First, we will need a slightly improved version of analyzer that will work just like the old one, but with one exception: it will not delete duplicate tokens. Instead, it will return all of the tokens found by its search.
fun TokenAnalyzer.analyze_betterTokenizingWithDuplicates(input: String): Collection<String> =
betterTokenizer
.tokenize(input)
.flatMap { lowerCaseFilter.filter(it) }
.flatMap { stopwordsFilter.filter(it) }
.flatMap { stemmingFilter.filter(it) }
.flatMap { synonymsFilter.filter(it) }
.sorted() // (1)
-
instead of
.sortedSet()
we just use.sorted()
so it gives us a list that potentially contains duplicate terms.
The tokenizer method we just created must now be used in the preparation of the new inverted index.
fun createIndexWithDuplicates() {
// delete old index
File(config.getInvertedIndexFile()).delete()
index.clear()
// generate new index
documentService.findAllIds().forEach { docId ->
documentService.findById(docId)?.let { doc ->
analyzer
.analyze_betterTokenizingWithDuplicates(doc) // (1)
.map { it to docId }
.forEach {
index[it.first]?.add(it.second)
?: index.put(it.first, mutableListOf(it.second))
}
}
}
File(config.getInvertedIndexFile()).writeText(gson.toJson(index))
}
-
the method differs from old
createIndex()
only by calling another analyzer.
We will also define a new indexing command that reuses the “duplicate terms” in our new inverted index.
fun createIndex(...) {
...
IndexType.INVERTED_DUPLICATED -> invertedIndexer.createIndexWithDuplicates()
...
}
Now, if we created a new index and inspect its contents, we can see that the posting lists now contain duplicate document IDs. We would expect this. The count of the duplicate IDs will correspond to the number of times the term appears in the given document.
shell:>create-index --type INVERTED_DUPLICATED
Index created: INVERTED_DUPLICATED
"left": [
"The Six Swans.txt",
"The Six Swans.txt",
"The Six Swans.txt",
"The Six Swans.txt",
"The Blue Mountains.txt",
"The Blue Mountains.txt",
"The Blue Mountains.txt",
"The Blue Mountains.txt",
"The Blue Mountains.txt",
"The Blue Mountains.txt",
"The Flower Queen's Daughter.txt",
"The Glass Mountain.txt",
"The Glass Mountain.txt",
"The Nightingale.txt",
"The Nightingale.txt",
"The Nightingale.txt",
"Mr. Lismore and the Widow.txt",
"Mr. Lismore and the Widow.txt",
"Mr. Lismore and the Widow.txt",
"Mr. Lismore and the Widow.txt",
"Mr. Lismore and the Widow.txt",
"Mr. Lismore and the Widow.txt",
"Mr. Lismore and the Widow.txt",
"The Witch.txt",
"The Witch.txt",
. . .
But now we also need a new search method. It will reuse the inverted index with duplicates and sort our results according to the count of search terms found in each document.
fun findWithScoring(request: String): Collection<String> =
analyzer
.analyze_betterTokenizing(request) // (1)
.asSequence()
.map { invertedIndexer.getPostingListByToken(it) }
.reduce { a, b -> a.plus(b) }
.asSequence()
.groupBy { it }
.map { it.key to it.value.size } // (2)
.sortedByDescending { it.second } // (3)
.map { it.first } // (4)
.toList()
-
For user queries it’s still possibly to use the old analyzer, which removes duplicates.
-
We do not need duplicate terms themselves, but only their number.
-
Sort so that the largest number takes the term up the list.
-
As an output, we are only interested in the terms themselves, which are already sorted in the correct order.
Of course, to use the new search method, we will also need a new command.
fun search(...) {
...
SearchType.SCORING -> addHeader(searchService.findWithScoring(query))
...
}
Okay. We’re ready to see how our new search method works.
shell:>search --type SCORING --query "massive glass peak"
Results found: 27
---------------------------------------
The Glass Mountain.txt
The Blue Mountains.txt
The Grateful Beasts.txt
The Iron Stove.txt
The Glass Axe.txt
The Story of Big Klaus and Little Klaus.txt
The Invisible Prince.txt
The Donkey Cabbage.txt
Mr. Lismore and the Widow.txt
A Story about a Darning-needle.txt
The Flower Queen?s Daughter.txt
The Wrong Black Bag.txt
Minions of the Moon.txt
Thumbelina.txt
The Three Strangers.txt
Alphege, or the Green Monkey.txt
The Little Green Frog.txt
In the Land of Souls.txt
The Witch and her Servants.txt
The Nightingale.txt
Blockhead Hans.txt
The Magic Ring.txt
The Four-fifteen Express.txt
Fairer-than-a-Fairy.txt
The Golden Crab.txt
The Seven-headed Serpent.txt
How Six Men travelled through the Wide World.txt
---------------------------------------
shell:>
As you can see, the number of documents in our results set is still 27, but now the documents are displayed with those having greater relevance at the top of the list. And, in order for the users to get to their desired results, they don’t need to go through the entire list—just the first few documents will likely be what they are seeking.
With this result, our system is ready to use, just as we intended, and it’s time to move on to the conclusions we might draw from this tutorial.
Tutorial Conclusion
We’ve made it! We’ve come to the end of our story.
Sure. Our final search algorithm is still not perfect, allowing us to search only in a very narrow range of possibilities. Nevertheless, it successfully demonstrates all the necessary components that are used to perform full-text searches. Let’s review all the evolutionary steps one more time so that we can clearly see once again the important concepts we should take away from this writing.
We began with a simple idea to look for the occurrence of strings in the source text. But it quickly became clear that the effectiveness of this method was extremely low. Nevertheless, we were introduced to the concept of a Boolean Search, which is the basis of all modern search engines.
In our next step, in order to improve our search results, we learned how to break the text into basic components, called tokens. We learned the process itself is called Tokenization. Precisely how the input text will be divided into tokens depends on the implementation of a particular tokenization algorithm, and this process may vary depending upon the task for which the tokens are being prepared.
Next, in order to unify our tokens, we introduced a process called Normalization. Normalization consisted of several transformations of tokens intended to make the search process more natural and easier to use.
During the normalization process, we first brought tokens into one register, then filtered our token list, removing all Stop Words. Stop words are those words that occur frequently in the documents but are also words which carry no semantic value and do not define the content of the documents.
We also applied Stemming, the process of modifying tokens and assigning their root shape (i.e., their stem form). Finally, in our normalization process, we enriched our tokens with Synonyms.
Then, when looking for ways to accelerate our search process, we moved away from the concept of enumerating documents and their indexes. Instead, we introduced a new concept: a search using an Inverted Index.
Last, we added a function to calculate the ranking of documents. The method we used was to sort the documents based on their Relevance to the search query, displaying the more relevant documents at the top of the list for our users.
These concepts are not unique nor invented by us for use in our search engine. You will be meeting them repeatedly when you use real search engines such as Elasticsearch or Solr. And we hope that, after reading this tutorial, these concepts will be more understandable to you, reducing the challenges you face when using them.
Happy searching!